Markov models
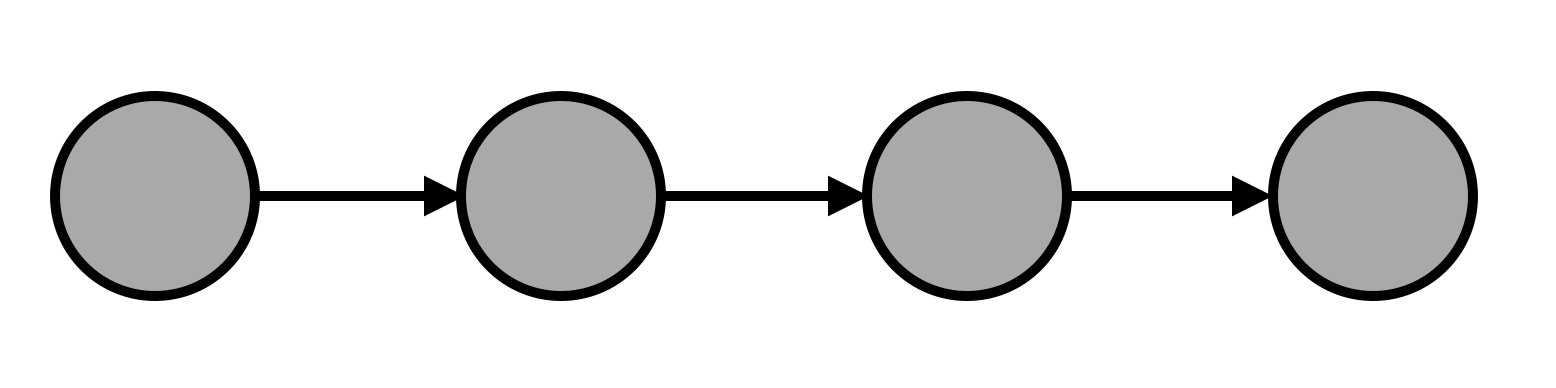
Sequences are all over the place. Football games are a sequence of plays, DNA is a sequence of amino acids, and sentences are sequences of words. Having a probabilistic model of how sequences arise can allow you to predict elements that haven’t seen yet. For example:
Autocomplete, helping us ask the most important questions first.
Markov models assume that all you need to know to predict an element sequence is which element came before it.
Sampling from the discrete distribution
Taking a single sample:
discrete([1/6, 2/6, 1/6, 1/6, 0.5/6, 0.5/6])
Looking at a histogram of 1000 samples:
// look at histogram of 1000 samples
hist(repeat(1000,
function() { discrete([1/6, 2/6, 1/6, 1/6, 0.5/6, 0.5/6]) }))
Using samples from discrete
to access elements of an array of words:
var words = ["^", "complete", "the", "sandwich", "sentence", "$"];
words[ discrete([1/6, 2/6, 1/6, 1/6, 0.5/6, 0.5/6]) ]
Word salad: building sentences just by repeat
ing calls to discrete
.
repeat(10,
function() { return words[ discrete([1/6, 2/6, 1/6, 1/6, 0.5/6, 0.5/6]) ] }).join(" ")
These are awful sentences! Note the predominance of the more frequent words.
Transitioning from one word to another
Defining a transition matrix:
var T = {
// transition probabilities from current word to
// current word ^ complete the sandwich sentence $
"^": [ 0, 0.5, 0.3, 0.1, 0.1, 0 ],
"complete": [ 0, 0, 0.6, 0.2, 0.1, 0.1 ],
"the": [ 0, 0, 0, 0.6, 0.4, 0 ],
"sandwich": [ 0, 0, 0, 0, 0, 1 ],
"sentence": [ 0, 0, 0, 0, 0, 1 ]
};
Defining a function that transitions from one word to the next:
var transition = function(word) {
var transitionProbabilities = T[word];
var sampledWordIndex = discrete(transitionProbabilities);
return words[sampledWordIndex];
}
Recursively transitioning to build up a sentence
var _sampleSentence = function(wordsSoFar) {
var prevWord = wordsSoFar[wordsSoFar.length - 1];
if (prevWord == "$") {
return wordsSoFar;
}
var nextWord = transition(prevWord);
return _sampleSentence( wordsSoFar.concat(nextWord) );
}
var sampleSentence = function() {
return _sampleSentence(["^"]).slice(1,-1).join(" ");
}
hist(repeat(1000, sampleSentence))
Application: predicting the next part of the sentence (autocomplete)
var predictNextPart = function(prefix) {
var words = prefix.split(" ");
var lastWordOfPrefix = _.last(words);
// q: why don't you need to seed the chain with the last word of the prefix?
// why not the *entire* prefix?
return _sampleSentence([lastWordOfPrefix]).slice(1,-1).join(" ");
}
repeat(10, function() { return predictNextPart("complete the") })
Application: learning the autocomplete transition probabilities from a corpus
for large applications, it’s infeasible to declare the transition matrix by hand. you want to learn it from data.
(we’re using dirichlet as a distribution on distributions)
var vocab = "^ $ factory 500 cookie fortune".split(" ");
var n = vocab.length;
var dirichletParams = repeat(n - 1, function() { return 1 });
var model = function() {
// transition matrix: represented as dictionary
// a key is a single vocab word; its corresponding value
// is an array of transition probabilities
var T = _.object(map(
function(w) {
// don't need to worry about transitions for $
if (w == "$") {
return [w, []];
}
// prevent transitions to ^
return [w, [0].concat(dirichlet(dirichletParams))];
},
vocab));
// given a current word, sample the next word
var transition = function(word) {
var probs = T[word];
return vocab[discrete( probs )];
}
// based on the last entry in a list of words,
// use the transition function to append a next word
var _predict = function(words) {
var prevWord = _.last(words);
if (prevWord == "$") {
return words;
}
return _predict(words.concat(transition(prevWord)));
}
// convenience method for predicting
var predict = function(string) {
return _predict(("^ " + string).split(" "))
.join(" ")
.replace("^","")
.replace("$","");
}
var _observe = function(wordsLeft, wordsSoFar) {
var prevWord = _.last(wordsSoFar);
var nextWord = wordsLeft[0];
if (wordsLeft.length == 0 | prevWord == "$") {
return wordsSoFar;
}
// sample the next word
var sampledNextWord = transition(prevWord);
factor(sampledNextWord == nextWord ? 0 : -100);
return _observe(wordsLeft.slice(1), wordsSoFar.concat(sampledNextWord));
}
var observe = function(string) {
var words = (string + " $").split(" ");
return _observe(words, ["^"]) // don't have to observe ^
.join(" ")
.replace("^","")
.replace("$","");
}
observe("fortune cookie");
observe("fortune cookie");
observe("fortune cookie factory");
observe("fortune cookie factory");
observe("fortune cookie factory");
observe("fortune 500");
return predict("cookie").split(" ").slice(0, 3).join(" ")
};
print(ParticleFilter(model, 5000));
(Talk about closed form solution for max-likelihood transition params; PPL as a prototyping tool.)